Introduction
Clinical genomics embodies a paradigm-shifting transformation to health service practice and delivery over many circumstances and stages of life. The era of technology has led to the development of next-generation sequencing (NGS) methods that are highly applicable to clinical studies. Sequencing methodologies have enabled us to evaluate genome, transcriptome, and epigenome. The NGS technologies supplemented with improving algorithms for analysis are advantageous in clinical study and research including neurobiology, chronic illness, and cancer. With the pace at which NGS is flourishing, the era of precision medicine is not far away.Â
Some of the important technologies for clinical genomics with their associated challenges are listed below:
Single-Molecule Sequencing (Third Generation Sequencing)
For single-molecule sequencing, both PacBio RS and the MinIONâ„¢ nanopore sequencer provide sequence reads with longer lengths on the order of kilobases or tens of kilobases. PacBio sequencer was developed by pacific biosciences and has an error rate of 86% but repeated sequencing of each of the strands results in 99% accuracy. Also, many bioinformatics tools have been developed in order to deal with the error rate.Â
On the other hand, the MinIONâ„¢ sequencer distributed by Oxford Nanopore Technologies (ONT) is highly portable and has a relatively small cost. Nanopore technology is still at early developmental stages and its features allow it to bring sequencing to clinics themselves, especially in remote locations.Â
The tools used for analysis in PacBio and ONT are given in Table 1:
Table 1: Bioinformatic tools in the Third-generation sequencing with applications
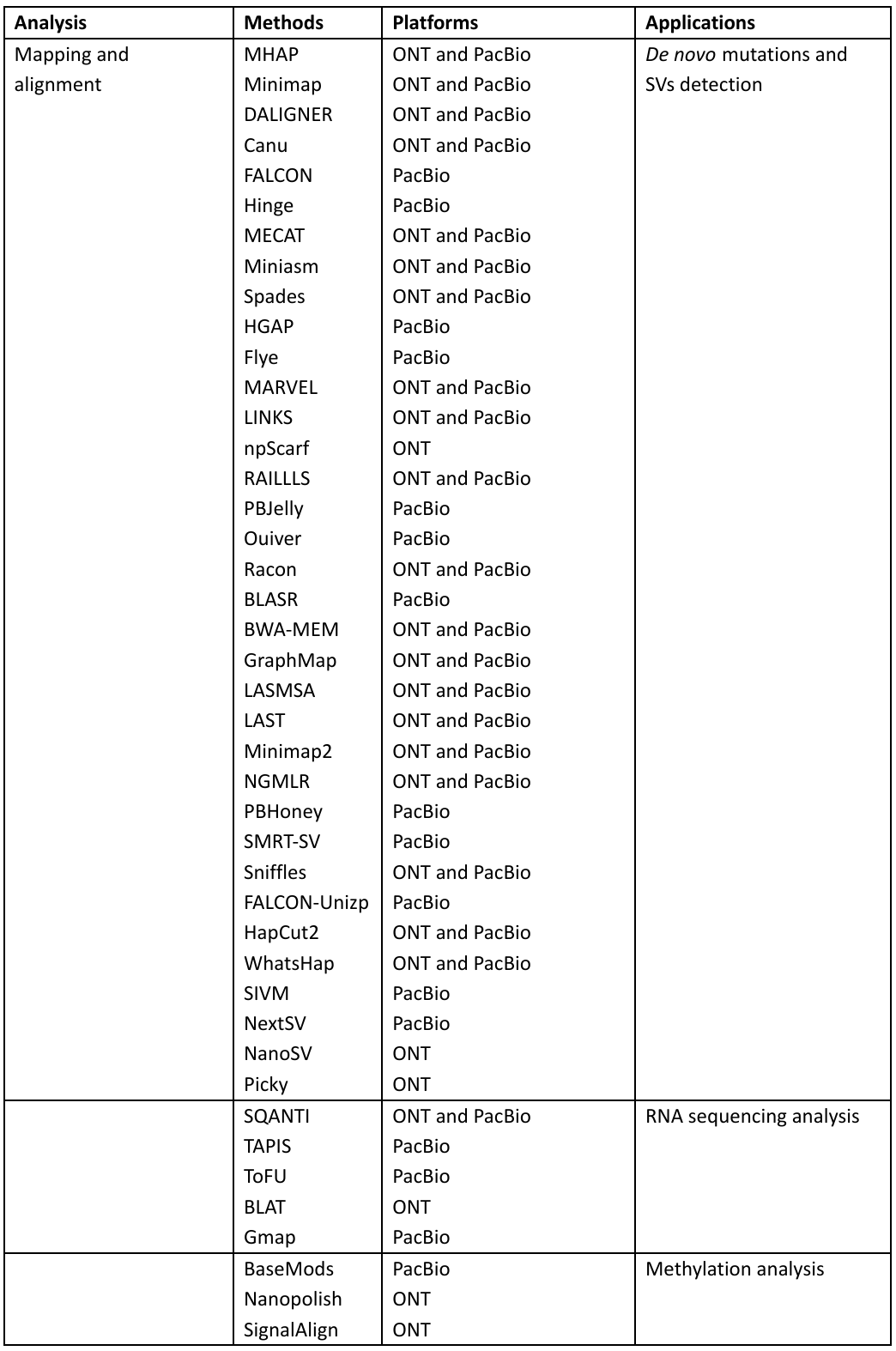
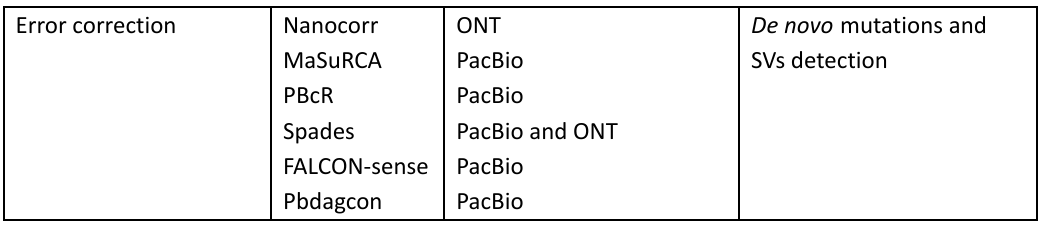
MinIONâ„¢ has demonstrated its potential in clinical study and research by focusing on the identification and characterization of the pathogens, it also includes sequencing of influenza virus, antibiotic resistance genes in salmonella typhi, and even Ebola virus for studying the transmission patterns.
DNA Sequencing for Clinical Applications
Many researchers have devoted their research in order to standardizing DNA sequencing. For any type of sequencing technology, two key factors are required, that is, accuracy and reproducibility of results. These requirements are also necessary for any type of clinical study. Through DNA sequencing, we are able to detect and identify somatic and germline mutations. The DNA sequencing can be categorized into whole-genome sequencing (WGS) and whole-exome sequencing (WES)
In the WGS approach, the complete DNA sequence of the genome is determined with a single assay. It is quite helpful in detecting single nucleotide variation (SNV). On the other hand, WES sequence only exonic regions, and this makes it a more cost-effective method to detect mutations and alternations. Its accuracy is high for the detection of indels but as WGS has high coverage as compared to WES, copy number variation (CNV) is limited in WES.Â
There is another more targeted and affordable method than WES. In this method, specific cancer panels are most commonly used and require prior information regarding recurrent genetic and epigenetic lesions. In many cancer types, recurrent somatic mutations appear and they can predict the risk level associated with the disease. For instance, in acute myeloid leukemia (AML), approximately 15 biomarkers are used to stratify patients who are previously put in the risk group at the intermediate level by cytogenetic classification. This method allows the construction of a treatment plan for risk-defined AML patients.Â
Thus, targeted sequencing gives us a deeper knowledge of known genes and also hotspots for mutation. Besides, with a decrease in the cost of WES, its potential is growing in clinical diagnosis and prognosis.Â
The technology of RNA Sequencing (RNA-seq)
Whole transcriptome analysis can be done through RNA-sequencing technology. It allows the detection of gene expression, fusion genes, alternative isoforms, and expressed variants. But besides all these benefits, this technology is quite sensitive to systematic bias. There are a number of software packages that are useful for gene expression normalization. EDAseq is the tool that corrects both quantification bias and intra-group variations caused due to gene length and GC content, making this technique more accurate for differential gene expression analysis.Â
Similarly, PEER and sva have great power in the detection of the latent variable for gene expression quantification among different sequencing data sites. For an RNA -seq study design with high statistical power, it is recommended to have consistent experimental strategies that include a sequencer. Read lengths, depth of sequencing, and most importantly the protocol. The high sequencing depth is important for discovering novel genes and also for accurate gene expression profiling. Also, by increasing the number of biological replicates, the accuracy of gene quantification is enhanced. Thus, RNA-seq study design is very important for precise analysis of differential gene expression.
DNA Methylation Provides a Complementary Approach to Clinical Measures for Patient Classification
DNA methylation is the addition of a methyl group to the 5th position of cytosine in human beings for suppressing gene expression. DNA methylation is the important hallmark of cancer and aging. The Cancer Genome Atlas (TCGA) consortium along with other research groups have determined that the classification of cancer can be based on the degree of DNA methylation. In many subgroups of cancer like breast cancer, gastric cancer, brain cancer etc. CpG island methylator phenotype (CIMP) exists. The patients with CIMP can also be used to classify and stratify patients into groups having different clinical results.Â
There are many benefits to doing clinical profiling through DNA methylation analysis:
- The analysis is not dependent on genetic alternations of disease but it can be equally applied to limited somatic mutations.
- The DNA is being analyzed which is beneficial as it is less sensitive to enzymatic degradation and heat in comparison to RNA which enables us to have more accurate profiling.
There are many methods for quantitative measurement of DNA methylation. They can be grouped into three categories:
- PCR-based technique: They are mostly utilized as a validation approach for high-throughput quantification.Â
- Microarray-based techniques: Among these approaches, HpaII tiny fragment enrichment through ligation-mediated PCR (HELP) assay is a methylation quantification method for clinical sample profiling and research. This method is based on restriction enzyme HpaII activity that has the ability to recognize and cut methylated CpG DNA sites. Besides this, Illumina Infinium BeadChip Kit is another method for methylation quantification. This BeadChip array platform makes use of two distinct bead types in order to quantify DNA methylation levels at the level of single cytosine.Â
- Sequencing-based techniques: These methods have the ability to give us regional quantification of DNA methylation at the level of single-base resolution. These approaches make use of bisulfite conversion sequencing, where the bisulfite changes unmethylated cytosine to uracil while keeping the methylated cytosine intact. Then, in the final sequencing readout analysis, the unmethylated cytosine show itself as thymine. CpG methylation levels are calculated for individual sites that are based on the percentage of reads having cytosine in a total number of mapped reads. These bisulfite methods consist of whole-genome bisulfite sequencing (WGBS), targeted methylation sequencing (TMS), and reduced representation bisulfite sequencing (RRBS). Among these approaches, WGBS needs high depth sequencing i.e., at least four reads need to cover each base in the whole genome for precise quantification. This allows the inclusion of sites having low and high CG density. The RRBS and TMS approaches cover an only subset of the genome and they are cheaper alternatives to WGBS.Â
All of these targeted approaches allow profiling of patients with regions of interest in order to understand transcriptome regulation.Â
Besides this, regional quantification approaches are also present. These approaches mainly make use of affinity-based methylation sequencing like methylated DNA immunoprecipitation sequencing (MeDIP-seq). In this approach, genomic regions with methylation are identified by using antibodies. But still, more work needs to be done.
Computational Analysis of Multi-Omics Data
In any type of genomics analysis, the analysis of raw data produced as a result of sequencing or microarray analysis needs to be analyzed through the use of computational techniques. The main challenge is to analyze terabytes of data accurately and produce reproducible output. All the sequencing data analysis has multiple steps involved, but general steps in raw data analysis of sequencing include:
- Pre-processing of data, which involves a quality control step, and in this step faulty reads are removed from the analysis. Fast QC is the most common tool for this purpose.Â
- Alignment of reads to reference genome for detecting mutations and variations. Most commonly utilized tools include BWA for DNA reads, Bismark, BSMAP, or BSmapper for bisulfite sequencing data, and STAR for RNA-seq data. The choice of aligner depends upon the sequencing platform, read lengths, and SNP tolerance.Â
- After mapping, analysis is based on the scientific question for which the whole process is being done. For instance, through analysis of differential gene expression, variants and DNA methylation quantification can be done. For instance, Genome Analysis Toolkit (GATK) is used for variant calling for RNA-seq and DNA data, the output is then annotated by using Oncotator or SnpEff for cancer studies. Another important method for variant calling in cancer research is Pyclone. Besides these, for methylation data downstream analysis methylKit, eDMR, and methclone are used.
In cases where we have multiple types of data available, tools like iCluster and Cytoscape can be used to identify the individual and interacting processes taking part in the disease. No doubt, clustering of patients’ samples has allowed us to computationally combine different types of datasets and detect new subtypes that cannot be detected with a single type of data analysis. Still, there are lots of challenges in computational analysis like cost, technical standards, cross-platform, and analysis method but these developments in parallel sequencing methodologies have allowed us to have new opportunities in order to improve clinical research. Â
Despite challenges in cost, cross-platform comparisons, technical standards, and analysis methods, advances in massively parallel sequencing techniques present new opportunities to improve clinical research, which we explore in the next section.
Computational analysis for different sequencing technologies is given by figure 1:
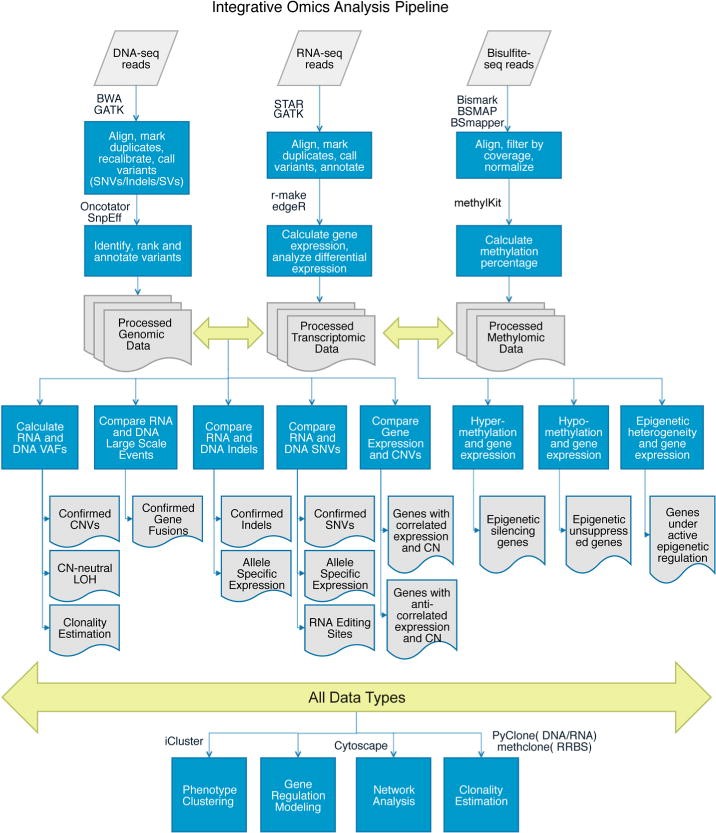
Figure 1: Computational analysis pipelines for integrative genomic, transcriptomic, and methylomic data.
Implementation and application in clinical research
The methodologies mentioned in the previous section have opened up lots of opportunities and research areas in the field of clinical genomics. Some of the important implementations are given by:
Electronic Health Records Data
In many dimensions of patient care, genomics and informatics are being integrated, especially transition to electronic health records (EHR). Although there is a recent transition to EHR, the use of machine learning and data mining was already being used to leverage data in large-scale studies. HER provides a source of large sample size and also diverse patient groups. This data can help in the mining of adverse effects of drugs and act as a classifier for disease phenotype relation.Â
Chronic Illnesses and Genomics
Chronic illness needs genomic approaches for prevention and management like diabetes and inflammatory bowel disease. The endeavors like metagenomic studies and the Human Microbiome Project have revealed the role of gut microbiota in health.Â
Personalized Healthcare
There are many statistical models present that can incorporate various genomic features and also family history together with weight, age, and ethnicity in order to predict disease risks in individuals. These models have been found quite important for early diagnosis in individuals who are susceptible to cardiovascular diseases and diabetes. There are many clinical genomics platforms like Personalis, Ingenuity, and Foundation Medicine that allow genetic testing in clinical platforms. There are also many direct-to-consumer tools like ancestry.com and 23and me which have made this sort of information in reach to concerned individuals. Also, in this way people are more aware of their health. There is still a lot of research is being carried out in disease risk prediction by using computational methods and it uses genetic information of patients together with EHR in some of the cases. The ethical and legal issues associated with clinical genomics are related to genomic testing in adolescents and children.
Cancer and Genomics
No doubt, genomics has faced many challenges in the field of medicine but with time it produced a paradigm shift, especially in the field of cancer treatment. Cancer was originally categorized on the basis of the type of tissue it affects but now it is being classified on the basis of genetic alternations. There is a great deal of knowledge that has been taken from the cancer sequencing efforts like the International Cancer Genome Consortium and The Cancer Genome Atlas. They have enhanced our understanding of the underlying genetic mechanisms, molecular subtypes, and cancer heterogeneity. The data is easily available to the scientific community and tools like the cBio Portal allow anyone to query the mutation load of any given gene in all assayed cancer types. Thus, in this way cancer genomics is being applied in a clinical setting.Â
An important success story in cancer therapy through genomics is related to metastatic melanoma in which BRAF is inhibited by vemurafenib. After the genomic screening of melanoma patients was done, BRAF V600 mutations were found in about half of the patients. This finding enabled to produce BRAF inhibitors, affecting cancer cells. One of the important challenges in targeted therapy is the development of resistance which also occurred in the patients with melanoma treated with BRAF inhibitors. The risk of resistance is reduced by using a combination of drugs in comparison to monotherapies. In this same example, when dabrafenib was used in combination with trametinib, progression-free survival was ensured, and also the response rates were enhanced as compared to monotherapy treatment of melanoma.Â
Thus, the combination therapies are performed with success as they reduced the resistance instances. Computational methods are used to predict the drug combinations and this decreases the cost of experimental testing in order to have a correct drug combination for every cancer model. In these methods, machine learning methods are used to take data from different cell line assays as training sets and then predict successful or potential combinations based on genetically-defined subtypes. Then these combinations can be tested by researchers on xenograft models. Some of the datasets that are being used in cancer research are NIH’s Library of Integrated Cellular Signatures (LINCS), NCI’s cancer cell line and drug screening data, and the Broad Institute’s connectivity map.Â
The efficacy of different types of chemotherapies or targeted therapies has been predicted by drug-gene interaction modeling coupled with genomic mutations present in a patient’s tumor. These methods contain not only rule-based decision-tree methods but also involve complex computational methods. Besides predicting the efficacy of the combination therapies, drug repositioning can also be predicted with computational methods for effective therapies.Â
These drug development and prediction methods depend on accurate and thorough stratification of patients’ genomic data. For this purpose, clinical samples undergo WES, WGS, and transcriptome sequencing either at the time of sample collection or after the banking has been done for future analysis. A large amount of sequencing data has allowed us to have a better evaluation of prognosis in many cases. Although, sequencing of the genome has enabled us in many important aspects of human health and disease but cancer detection and treatment remain to be the most important application. As cancers are the culmination of inherited and somatic genetic mutations, resulting in a heterogeneous subpopulation of cells. These subpopulations have been observed experimentally through cytogenetic, Sanger sequencing, and NGS. Genomics has allowed us to determine the composition of tumors and identify the molecular characteristics of different types of subpopulations.Â
The important challenge in this regard is accurately detecting heterogeneity and clonal evolution is the phenomena in most of the tumor profiling approaches that involves bulk of samples and effect in hiding the intratumoral variability. these subpopulations can now be measured with the help of single-cell sequencing technologies. Single cell sequencing has many important clinical applications in tumor sequencing as it enhances the resolution allowing us to model complex dynamics of tumor and then incorporate this knowledge in prognosis evaluation and prediction of drug efficacy (Figure 2). The single-cell sequencing methods have addressed the issues of heterogeneity and now researchers have ability to reveal new level of heterogeneity that cannot be detected in bulk samples. Also, this technique has proved that resolution at single cell level is necessary to characterize complex tissue samples.
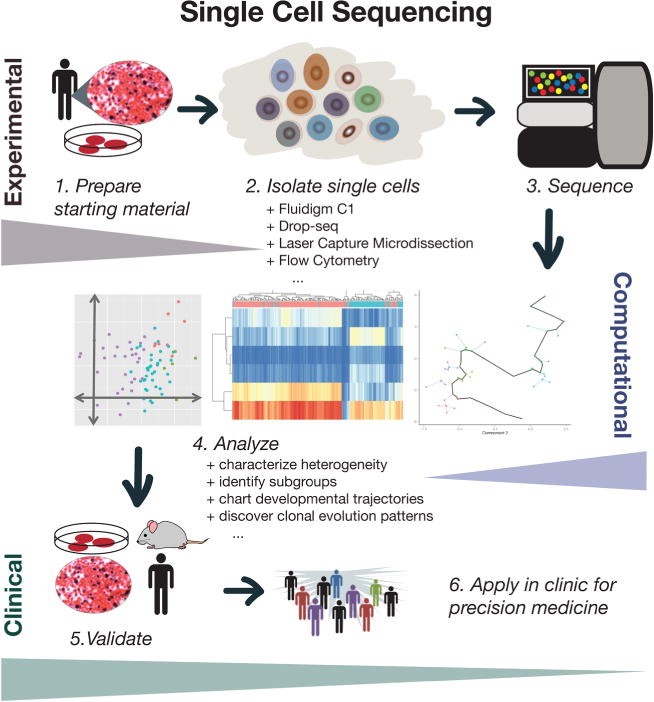
Figure 2: Theoretical overview of single-cell sequencing for clinical applications.
Besides all the above-mentioned importance and benefits, this sequence data can also be submitted in curated data repositories that includes database of Genotypes and Phenotypes, the Gene Expression Omnibus and the Sequencing Reads Archive. This public data availability will alleviate the issues like small sample size in clinical setting or in rare diseases.
Neurobiology and Genomics
The genomic sequencing technology for molecular stratification in order to predict and guide patient therapy is not limited to cancer drugs only. Although, neurobiology is not very deeply understood but genomic approaches are also applied to neurobiology, especially in the study of autism spectrum disorder and Alzheimer’s disease. There is a lot of effort and research being done to map the human brain by using cutting-edge brain imaging techniques, large volume data methods are becoming quite essential in neurodegenerative diseases. Understanding mutations and predispositions in these diseases will allow us to detect diseases at early stages and ameliorate their effect through drugs.
Future Perspective
No doubt, clinical genomics has opened a new window in clinical research. It allowed us to study human health and disease in a better light and most importantly the developments made in the field of oncology are quite remarkable. The future of clinical genomics in precision medicine for individuals. The development of drugs according to the genetic make-up of individuals is an important step forward. But this can be done by overcoming the challenges currently faced.